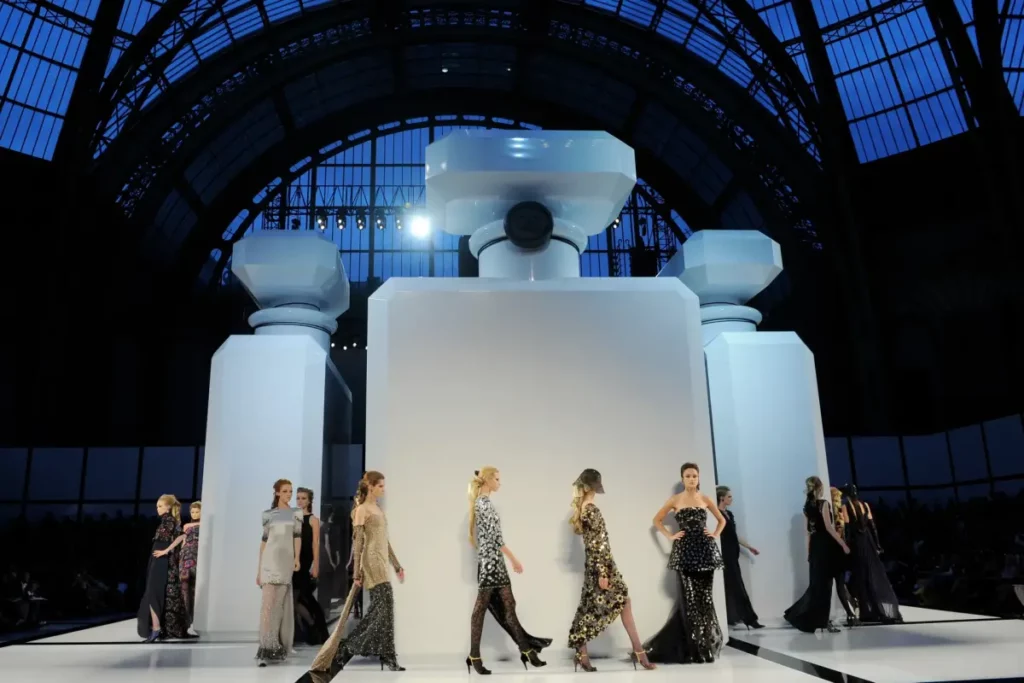
Fashion forecasting was initially a means of discussing fashion, but it evolved into a tool for staying ahead in the industry. This practice involves predicting societal and consumer moods, behaviors, and purchasing habits to anticipate future trends in the fashion business. Fashion trends often cycle every 20 years, and forecasting helps identify potential upcoming trends. The primary purpose of fashion forecasting is to keep up with current trends and foresee consumer desires, allowing brands to prepare in advance. Esteemed brands like Vogue and Gucci utilize this method to keep their designers informed about future developments in the fashion world.
Fashion forecasting has undergone a remarkable transformation over the years. In the past, it was largely driven by intuition and historical trends, with fashion experts relying on their experience and gut feelings to predict what consumers would want in the upcoming seasons. However, the advent of advanced data analytics and the integration of Product Lifecycle Management (PLM) systems have revolutionised the fashion industry, making fashion forecasting more precise, reliable, and efficient.
The Evolution of Fashion Trends Forecasting
Fashion brands and agencies are used to predict trends by analyzing runway shows, trade shows, newspapers, magazines, and market research. In the past, these were the only sources available for planning future collections. However, the fashion industry has evolved, and now prescriptive and predictive analytics complement the traditional methods.
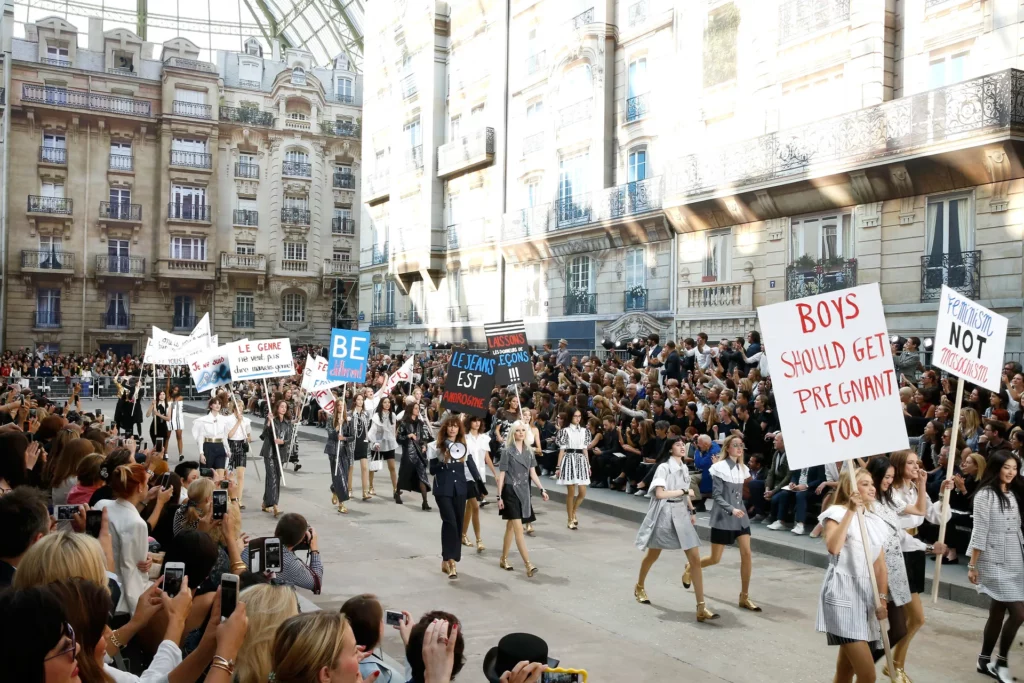
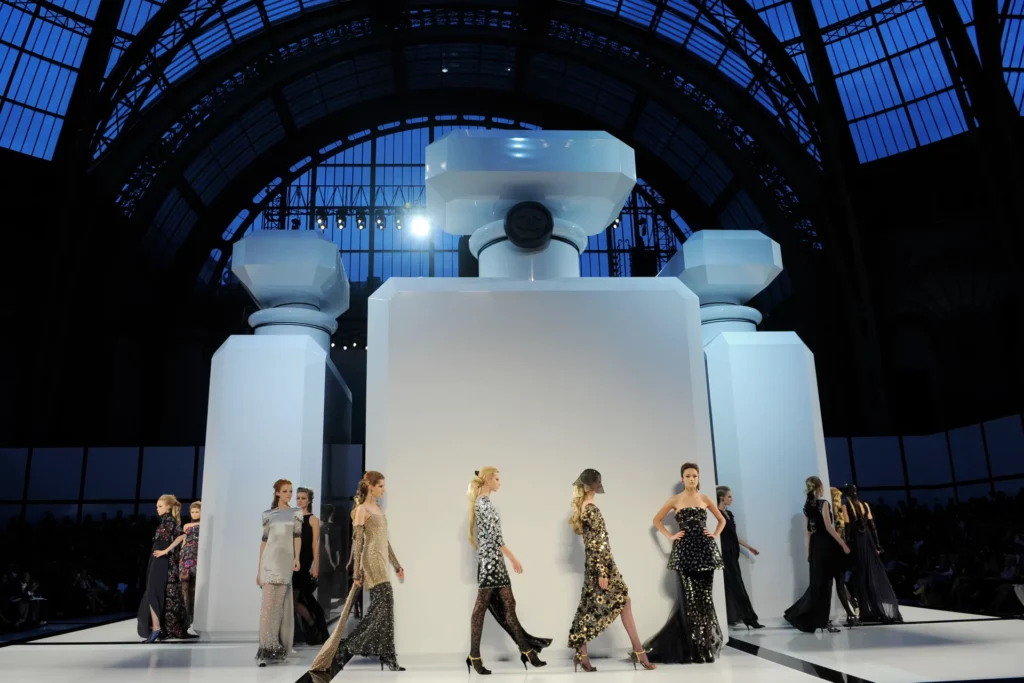
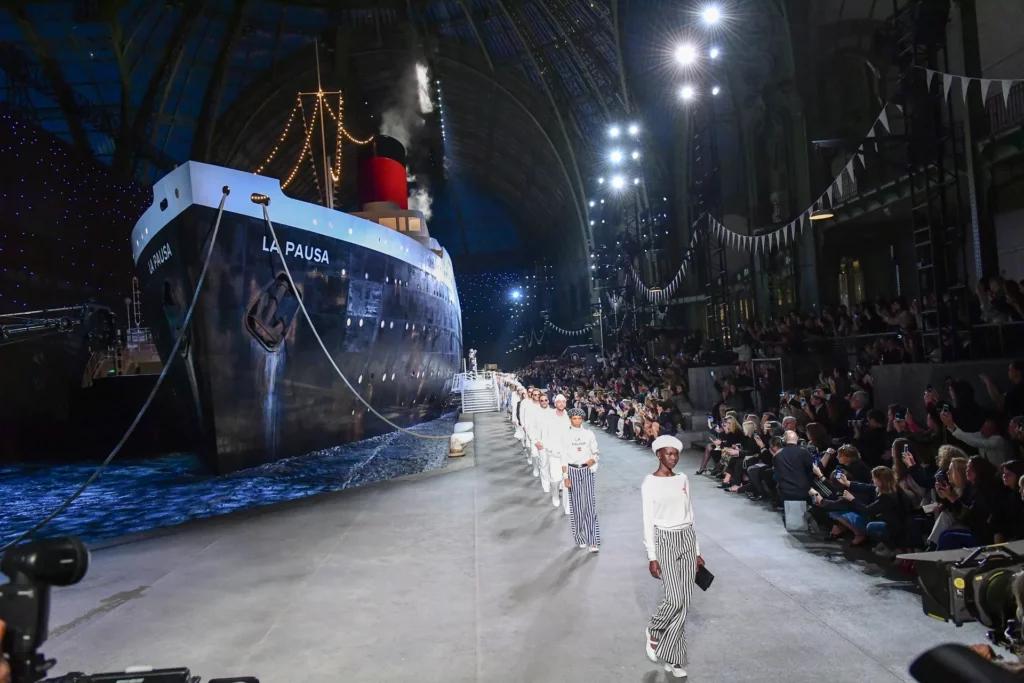
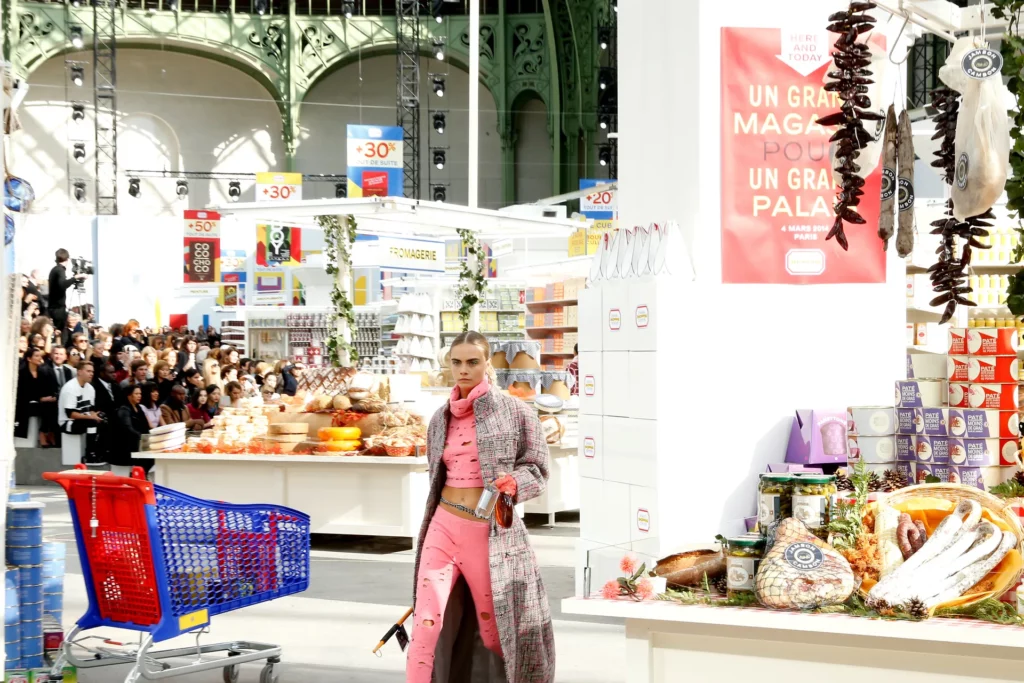
Fashion designers rely on trend forecasts to make informed decisions about upcoming styles and collections. Traditionally, fashion forecasting was an intricate blend of art and science. Experts would scrutinize various elements such as consumer behavior, market trends, cultural influences, and historical data. They would then use their experience and intuition to forecast future trends. This method, though effective to some extent, had its limitations. It was highly subjective and often failed to capture subtle shifts in consumer preferences and emerging trends. This could lead to missteps in design and production, resulting in financial losses and wasted resources.
Today, fashion forecasting has become a data-driven discipline. The integration of advanced data analytics allows fashion companies to leverage vast amounts of data to identify patterns, predict trends, and make informed decisions. This shift from intuition-based to data-driven forecasting has significantly enhanced the accuracy and reliability of trend predictions.
The Role of Data in the Modern Fashion Industry Forecasting
Data-driven fashion forecasting involves collecting and analyzing data from a wide range of sources. These sources include sales figures, social media interactions, online searches, and more. By analyzing this data, fashion companies can gain insights into consumer behavior, market trends, and cultural influences. Fashion forecasters analyze this data to predict upcoming trends and guide fashion companies in their design and production decisions. Here are some key aspects of data-driven fashion forecasting:
- Big Data Collection: The foundation of data-driven fashion forecasting is the collection of vast amounts of data. This includes sales data, social media posts, online searches, and other relevant information. Advanced technologies such as artificial intelligence and machine learning are used to gather and process this data efficiently.
- Data Analysis: Once the data is collected, it needs to be analyzed to identify patterns and trends. This involves using sophisticated algorithms and machine learning models to process the data and extract meaningful insights. These insights can reveal emerging trends, shifts in consumer preferences, and other valuable information.
- Predictive Analytics: The final step in data-driven fashion forecasting is predictive analytics. This involves using historical data and current trends to forecast future trends. Predictive analytics can help fashion companies anticipate consumer demand, plan production schedules, and make informed decisions about design and marketing strategies.
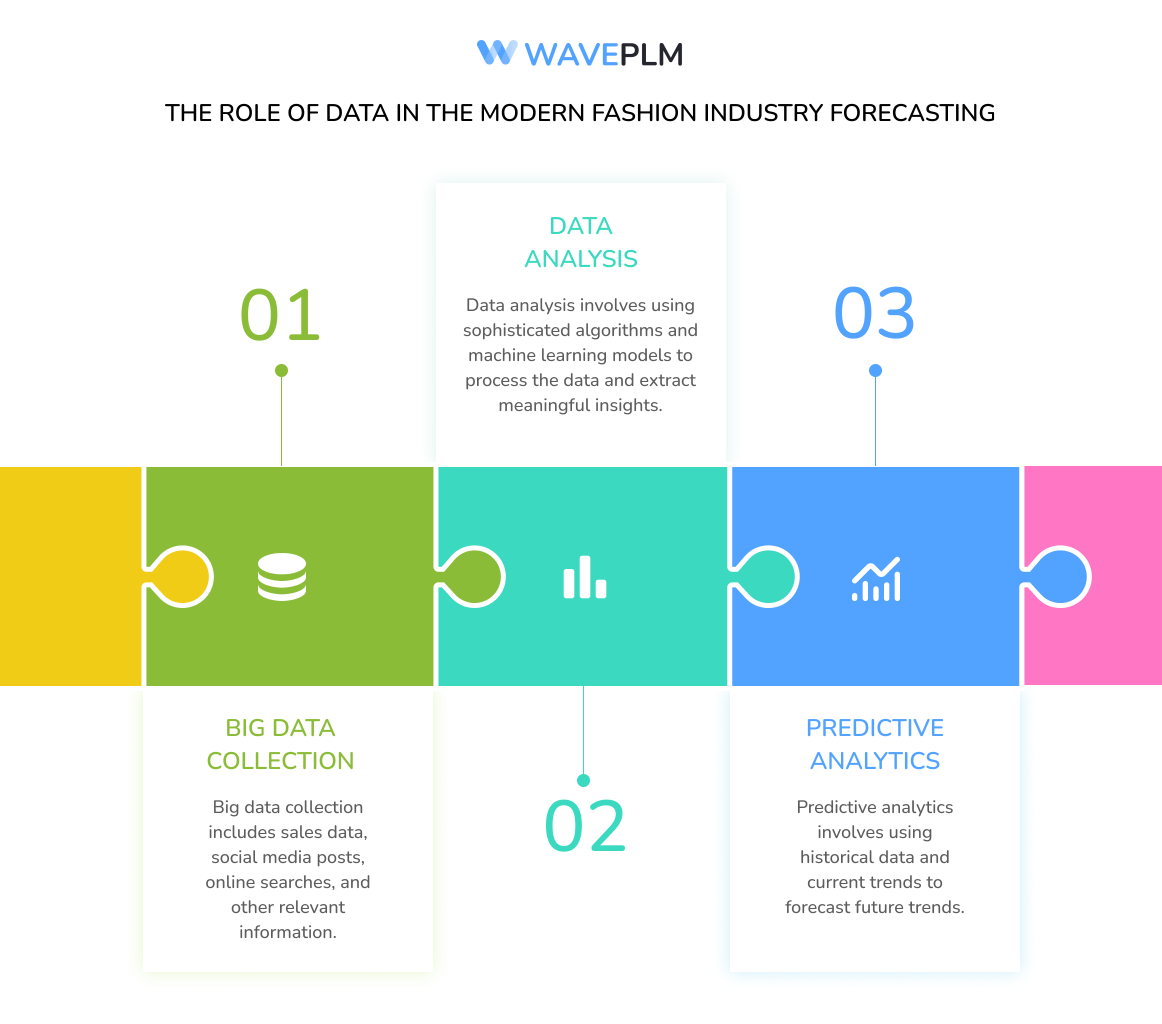
Integrating PLM with Data Analytics
Product Lifecycle Management (PLM) systems play a crucial role in modern fashion forecasting. PLM is a system that manages the entire lifecycle of a product, from its inception through design and manufacturing to its eventual disposal. An accurate fashion forecast is crucial for aligning production schedules and meeting consumer demand. By integrating PLM with data analytics, fashion companies can enhance their forecasting capabilities in several ways:
- Centralized Data Repository: PLM systems provide a centralized repository for all product-related data. This makes it easier to access and analyze data from different stages of the product lifecycle. By having all the data in one place, fashion companies can gain a comprehensive view of their products and make more informed decisions.
- Collaboration: PLM systems facilitate collaboration between different departments within a fashion company. This ensures that data insights are shared and utilized effectively across the organization. For example, designers can use insights from sales and marketing data to create products that align with consumer preferences.
- Real-Time Updates: PLM systems provide real-time updates on product development, production, and sales. This allows fashion companies to respond quickly to emerging trends and make adjustments to their strategies as needed. Real-time updates also enable better coordination between different departments, leading to increased efficiency and reduced time-to-market.
- Enhanced Accuracy: By combining PLM data with external data sources, fashion companies can enhance the accuracy of their forecasts. For example, they can use sales data from different regions to identify regional trends and adjust their production schedules accordingly. This helps to minimize the risk of overproduction and stock shortages.
Benefits of PLM-Enhanced Fashion Forecasting
Fashion trend forecasting plays a vital role in improving the design process and ensuring market responsiveness. The integration of PLM like Wave PLM with data analytics offers numerous benefits for fashion companies. Here are some of the key advantages:
- Improved Design Process: By using data insights, designers can create products that are more likely to resonate with consumers. This reduces the risk of unpopular designs and increases the chances of success in the market. Data-driven design also enables designers to stay ahead of trends and create innovative products that set new standards in the industry.
- Efficient Production: Data-driven fashion forecasting helps manufacturers plan production schedules based on accurate demand forecasts. This minimizes waste and reduces the risk of overproduction. Efficient production also leads to cost savings and improved profitability for fashion companies.
- Cost Savings: By avoiding overproduction and reducing markdowns, fashion companies can save significantly. Accurate forecasting enables them to produce the right amount of products to meet consumer demand, reducing the need for excessive inventory and costly markdowns.
- Market Responsiveness: With the ability to quickly adapt to changing trends, fashion companies can stay ahead of the competition. Data-driven insights allow them to respond to emerging trends in real time, ensuring that they are always in sync with consumer preferences.
- Sustainability: Accurate forecasting and efficient production contribute to sustainability in the fashion industry. By minimizing waste and reducing overproduction, fashion companies can reduce their environmental impact. This aligns with the growing demand for sustainable practices in the industry.
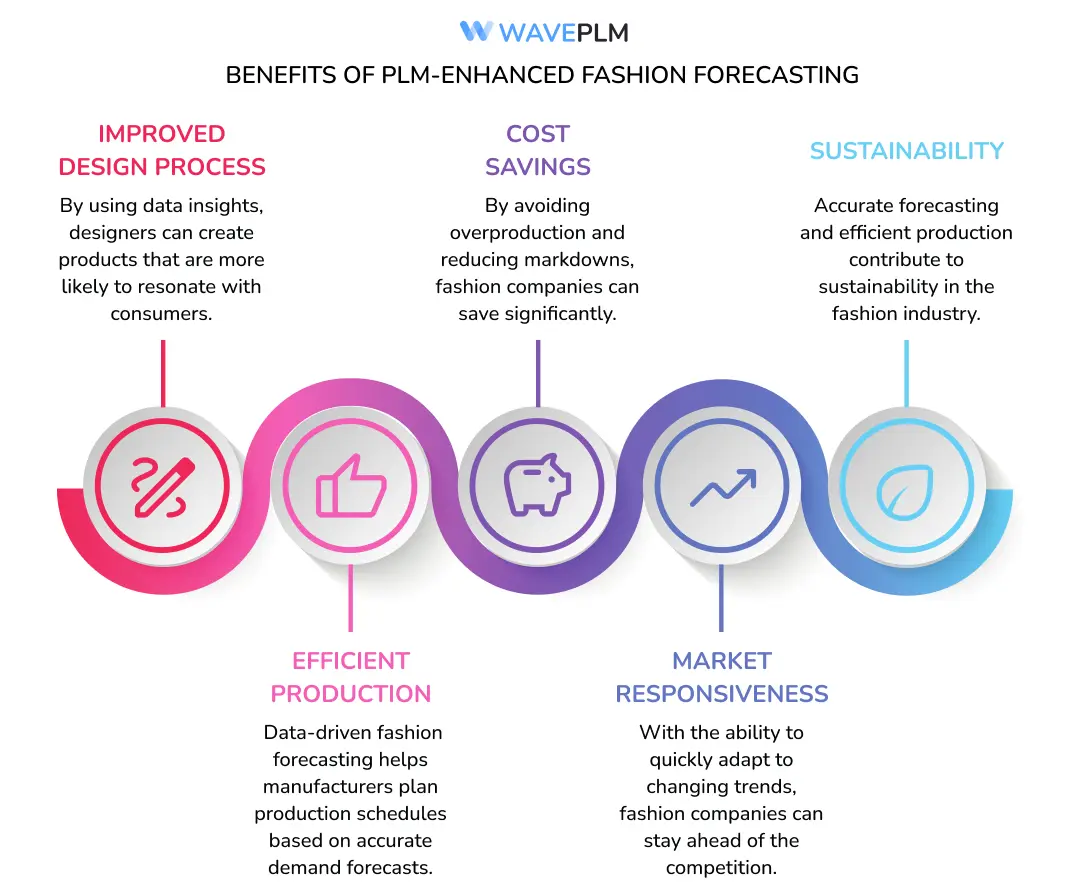
Case Studies: Successful Implementation of PLM-Enhanced Fashion Forecasting
To illustrate the benefits of PLM-enhanced fashion forecasting, let’s look at some case studies of fashion companies that have successfully implemented these systems. These companies have successfully used data analytics to forecast fashion trends and align their production schedules accordingly.
- Company A: This global fashion brand used Wave PLM and data analytics to streamline its design and production processes. By analyzing sales data and consumer feedback, they were able to identify emerging trends and create products that resonated with their target audience. This led to a 24 % increase in sales.
- Company B: Another fashion company, known for its innovative designs, leveraged PLM systems to enhance collaboration between its design, production, and marketing teams. By integrating data analytics into their PLM system, they could forecast demand more accurately and align their production schedules accordingly. This not only reduced waste and overproduction but also enabled them to respond swiftly to market changes, maintaining a competitive edge.
- Company C: This fast fashion retailer utilized PLM and big data analytics to optimize its supply chain. By analyzing real-time sales data and social media trends, they could predict which styles would become popular and quickly produce those items. This agility allowed them to meet consumer demand efficiently and reduce the time from design to retail, ensuring they always had the latest trends in stores.
The Future of Fashion Trend Forecasting
As technology continues to advance, the role of data in fashion forecasting will only grow. Emerging technologies such as artificial intelligence (AI), machine learning, and the Internet of Things (IoT) are poised to further enhance the capabilities of fashion forecasting. Trend forecasters will increasingly rely on AI and machine learning to identify complex patterns and predict future trends. Here’s a glimpse into the future of fashion forecasting with these technologies:
- Artificial Intelligence and Machine Learning: AI and machine learning algorithms can analyze vast amounts of data much faster and more accurately than humans. These technologies can identify complex patterns and correlations that might be missed by human analysts. In the future, AI-driven fashion forecasting tools will become more sophisticated, providing even more precise and actionable insights.
- Internet of Things (IoT): IoT devices, such as smart mirrors and connected garments, can provide real-time data on consumer preferences and behaviours. This data can be integrated into PLM systems to enhance forecasting accuracy. For example, smart mirrors in retail stores can track which items consumers try on but do not purchase, providing valuable insights into potential design improvements.
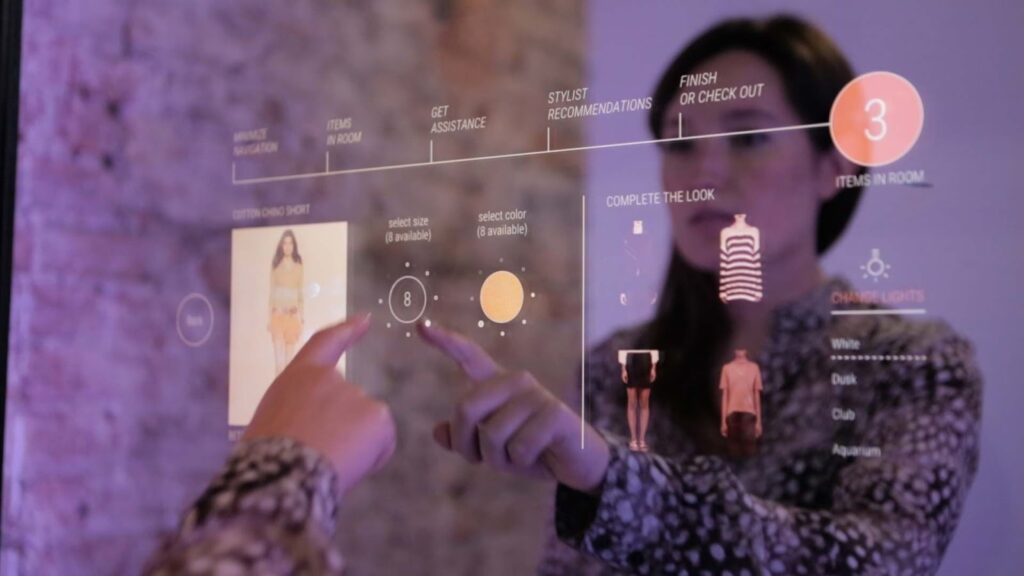
- Augmented Reality (AR) and Virtual Reality (VR): AR and VR technologies can transform the way fashion companies design and present their products. Virtual fitting rooms and AR-based product visualizations can help consumers make more informed purchasing decisions. This data can be collected and analyzed to understand consumer preferences better and predict future trends.
- Blockchain Technology: Blockchain can enhance transparency and traceability in the fashion supply chain. By providing a secure and immutable record of transactions, blockchain can ensure the authenticity of data used in fashion forecasting. This is particularly important for verifying the origin and sustainability of materials, which is becoming increasingly important to consumers.
Challenges and Considerations for Fashion Companies
While the integration of PLM and data analytics offers significant benefits, there are also challenges that fashion companies need to consider:
- Data Quality and Management: The accuracy of fashion forecasting depends on the quality of the data used. Fashion companies must ensure that they collect and manage high-quality data. This involves cleaning and standardizing data from various sources to ensure consistency and reliability.
- Privacy and Security: Collecting and analyzing consumer data raises concerns about privacy and security. Fashion companies must implement robust data protection measures to safeguard consumer information. Compliance with data privacy regulations, such as GDPR, is also essential.
- Integration and Implementation: Integrating PLM systems with data analytics tools can be complex and require significant investment. Fashion companies need to carefully plan and execute the implementation process to ensure a seamless integration. This may involve upgrading existing systems, training staff, and addressing any technical challenges that arise.
- Cultural Change: Adopting data-driven fashion forecasting requires a cultural shift within fashion companies. Decision-makers must embrace data-driven insights and be willing to rely less on intuition. This change in mindset can be challenging but is essential for the successful implementation of data-driven forecasting.
Conclusion
Integrating data insights with PLM systems transforms fashion forecasting from a subjective art into a precise science. By leveraging advanced data analytics, fashion companies can gain valuable insights into consumer behaviour, market trends, and cultural influences. This allows them to make better-informed decisions about design, production, and marketing, leading to increased efficiency, cost savings, and market responsiveness.
Trend reports provide actionable insights that help fashion companies stay ahead of market trends and consumer preferences.
The benefits of PLM-enhanced fashion forecasting are clear: improved design processes, efficient production, cost savings, market responsiveness, and sustainability. Fashion companies that embrace these innovations will be better positioned to stay relevant and competitive in a rapidly changing market.
As technology continues to evolve, the future of fashion forecasting looks promising. AI, machine learning, IoT, AR, VR, and blockchain are set to enhance the capabilities of fashion forecasting further, providing even more accurate and actionable insights. However, fashion companies must also address challenges related to data quality, privacy, integration, and cultural change to realize the potential of these technologies fully.
In conclusion, the combination of data analytics and PLM systems is revolutionizing fashion forecasting. By embracing these innovations, fashion companies can ensure they stay ahead of trends, meet consumer demand efficiently, and operate more sustainably. The future of fashion is data-driven, and those who leverage the power of data will thrive competitively and dynamically industry.
Leave a Reply